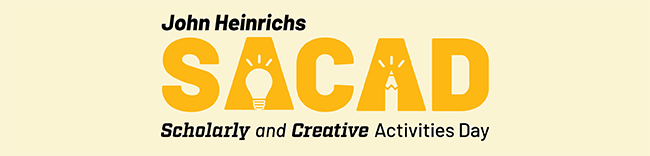
Abstract
Recent advances in machine learning (broadly) and random forest analysis (specifically) have allowed researchers to examine variables and datasets in previously unknown or unexplored ways. Following a recommendation by Fife and D’Onofrio (2022) to utilize machine learning in the social sciences, I used previously reported attraction data (Challacombe and Perdomo, 2021) on males who have sex with other males (MSM) and applied a random forest analysis against the variables. I also utilized two different programming languages—R and Python—to study the data. While both programming languages provided different data points, the general results were similar. In both studies, the importance of the top partner-specific variables remained about the same regardless of the programming language used—the variables of sexual position (in both studies) and dominance (in studies 1B and 2B) are important MSM attraction factors. This finding supports the original findings for this data. This study also showcases how novice researchers can utilize tools like machine learning and RF to conduct alternative analyses.
Department/Program
Psychology
Submission Type
online only poster
Date
3-27-2024
Rights
Copyright Challacombe 2024.
Recommended Citation
Challacombe, Darin
(2024)
"Attraction and Machine Learning: Evaluating MSM Attraction Preferences using Random Forest,"
SACAD: John Heinrichs Scholarly and Creative Activity Days: Vol. 2024, Article 111.
Available at:
https://scholars.fhsu.edu/sacad/vol2024/iss2024/111